Append a new batch of data#
We have one file in storage and are about to receive a new batch of data.
In this notebook, we’ll see how to manage the situation.
import lamindb as ln
import lnschema_bionty as lb
import readfcs
lb.settings.species = "human"
💡 loaded instance: testuser1/test-facs (lamindb 0.55.0)
hello
ln.track()
💡 notebook imports: anndata==0.9.2 lamindb==0.55.0 lnschema_bionty==0.31.2 pytometry==0.1.4 readfcs==1.1.7 scanpy==1.9.5
💡 Transform(id='SmQmhrhigFPLz8', name='Append a new batch of data', short_name='facs2', version='0', type=notebook, updated_at=2023-10-04 16:42:35, created_by_id='DzTjkKse')
💡 Run(id='Xw5xc4OteUjTU31pfcZC', run_at=2023-10-04 16:42:35, transform_id='SmQmhrhigFPLz8', created_by_id='DzTjkKse')
hello
within hello
Ingest a new file#
Access
#
Let us validate and register another .fcs
file from Oetjen18:
filepath = readfcs.datasets.Oetjen18_t1()
adata = readfcs.read(filepath)
adata
AnnData object with n_obs × n_vars = 241552 × 20
var: 'n', 'channel', 'marker', '$PnR', '$PnB', '$PnE', '$PnV', '$PnG'
uns: 'meta'
Transform: normalize
#
import anndata as ad
import pytometry as pm
pm.pp.split_signal(adata, var_key="channel")
pm.pp.compensate(adata)
pm.tl.normalize_biExp(adata)
adata = adata[ # subset to rows that do not have nan values
adata.to_df().isna().sum(axis=1) == 0
]
adata.to_df().describe()
CD95 | CD8 | CD27 | CXCR4 | CCR7 | LIVE/DEAD | CD4 | CD45RA | CD3 | CD49B | CD14/19 | CD69 | CD103 | |
---|---|---|---|---|---|---|---|---|---|---|---|---|---|
count | 241552.000000 | 241552.000000 | 241552.000000 | 241552.000000 | 241552.000000 | 241552.000000 | 241552.000000 | 241552.000000 | 241552.000000 | 241552.000000 | 241552.000000 | 241552.000000 | 241552.000000 |
mean | 887.579860 | 1302.985717 | 1221.257257 | 877.533482 | 977.505533 | 1883.358298 | 556.687953 | 929.493316 | 941.166747 | 966.012244 | 1210.769935 | 741.523184 | 1003.064857 |
std | 573.549695 | 827.850302 | 672.851319 | 411.966073 | 584.217139 | 932.113729 | 480.875917 | 795.550133 | 658.984751 | 456.437094 | 694.622980 | 473.287558 | 642.728024 |
min | 0.000000 | 0.000000 | 0.000000 | 0.000000 | 0.000000 | 0.000000 | 0.000000 | 0.000000 | 0.000000 | 0.000000 | 0.000000 | 0.000000 | 0.000000 |
25% | 462.757715 | 493.413744 | 605.463427 | 588.047798 | 495.437303 | 1063.670965 | 240.623098 | 404.087640 | 477.932659 | 592.294399 | 575.401173 | 380.247262 | 475.108131 |
50% | 774.350833 | 1207.624048 | 1110.367681 | 782.939692 | 782.981430 | 1951.855099 | 484.355203 | 557.904360 | 655.909639 | 800.280049 | 1124.574275 | 705.802991 | 775.101973 |
75% | 1327.792103 | 2036.849496 | 1721.730010 | 1070.479036 | 1453.929567 | 2623.975657 | 729.754419 | 1345.771633 | 1218.445208 | 1347.042403 | 1742.288464 | 1069.175380 | 1420.744291 |
max | 4053.903716 | 4065.495666 | 4095.351322 | 4025.827267 | 3999.075551 | 4096.000000 | 4088.719985 | 3961.255364 | 3940.061146 | 4089.445928 | 3982.769373 | 3810.774988 | 4023.968008 |
Validate cell markers
#
Let’s see how many markers validate:
validated = lb.CellMarker.validate(adata.var.index)
hello
❗ 9 terms (69.20%) are not validated for name: CD95, CXCR4, CCR7, LIVE/DEAD, CD4, CD49B, CD14/19, CD69, CD103
Let’s standardize and re-validate:
adata.var.index = lb.CellMarker.standardize(adata.var.index)
validated = lb.CellMarker.validate(adata.var.index)
hello
hello
hello
❗ 7 terms (53.80%) are not validated for name: CD95, CXCR4, LIVE/DEAD, CD49B, CD14/19, CD69, CD103
Next, register non-validated markers from Bionty:
records = lb.CellMarker.from_values(adata.var.index[~validated])
ln.save(records)
hello
❗ did not create CellMarker records for 2 non-validated names: 'CD14/19', 'LIVE/DEAD'
Manually create 1 marker:
lb.CellMarker(name="CD14/19").save()
hello
❗ record with similar name exist! did you mean to load it?
id | synonyms | __ratio__ | |
---|---|---|---|
name | |||
Cd14 | roEbL8zuLC5k | 90.0 |
Move metadata to obs:
validated = lb.CellMarker.validate(adata.var.index)
adata.obs = adata[:, ~validated].to_df()
adata = adata[:, validated].copy()
hello
❗ 1 term (7.70%) is not validated for name: LIVE/DEAD
Now all markers pass validation:
validated = lb.CellMarker.validate(adata.var.index)
assert all(validated)
hello
Register
#
modalities = ln.Modality.lookup()
features = ln.Feature.lookup()
efs = lb.ExperimentalFactor.lookup()
species = lb.Species.lookup()
markers = lb.CellMarker.lookup()
hello
hello
hello
hello
hello
file = ln.File.from_anndata(
adata,
description="Oetjen18_t1",
field=lb.CellMarker.name,
modality=modalities.protein,
)
Show code cell output
... storing '$PnR' as categorical
... storing '$PnE' as categorical
... storing '$PnV' as categorical
... storing '$PnG' as categorical
hello
hello
hello
hello
❗ 1 term (100.00%) is not validated for name: LIVE/DEAD
❗ no validated features, skip creating feature set
file.save()
file.labels.add(efs.fluorescence_activated_cell_sorting, features.assay)
file.labels.add(species.human, features.species)
hello
within hello
hello
within hello
hello
within hello
file.features
hello
hello
within hello
hello
within hello
hello
within hello
hello
within hello
Features:
var: FeatureSet(id='5VLdJw9hszLvo8xwC7Ny', n=12, type='number', registry='bionty.CellMarker', hash='fUG1EYvGEKSvt_GuvNaA', updated_at=2023-10-04 16:42:41, modality_id='Q6B6XiyC', created_by_id='DzTjkKse')
'CD14/19', 'CD8', 'Cd4', 'Ccr7', 'CD103', 'CD95', 'CD69', 'CD49B', 'CXCR4', 'CD27', 'CD45RA', 'CD3'
external: FeatureSet(id='eA6ogIIilUdLyVfVA9HN', n=2, registry='core.Feature', hash='qV1hwXGKngdbVta23Anl', updated_at=2023-10-04 16:42:41, modality_id='zBZgVlDk', created_by_id='DzTjkKse')
🔗 assay (1, bionty.ExperimentalFactor): 'fluorescence-activated cell sorting'
🔗 species (1, bionty.Species): 'human'
View data flow:
file.view_flow()
hello
within hello
hello
within hello
hello
within hello
hello
within hello
hello
hello
hello
hello
hello
hello
hello
within hello
hello
within hello
hello
within hello
hello
within hello
hello
hello
hello
hello
hello
Inspect a PCA fo QC - this dataset looks much like noise:
import scanpy as sc
sc.pp.pca(adata)
sc.pl.pca(adata, color=markers.cd8.name)
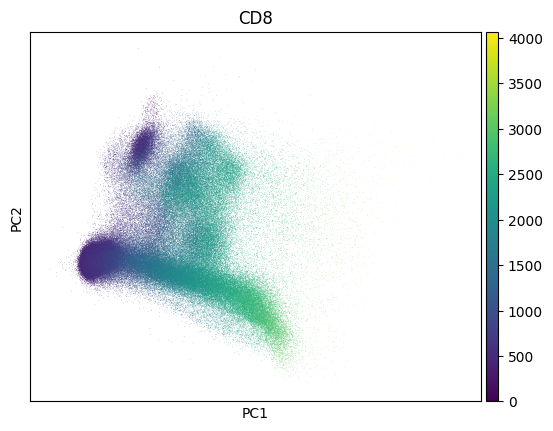
Create a new version of the dataset by appending a file#
Query the old version:
dataset_v1 = ln.Dataset.filter(name="My versioned cytometry dataset").one()
dataset_v2 = ln.Dataset(
[file, dataset_v1.file], is_new_version_of=dataset_v1, version="2"
)
hello
hello
within hello
hello
within hello
hello
within hello
hello
within hello
hello
hello
hello
hello
hello
dataset_v2
Dataset(id='dwdXtuUm7KR2XuBNSbsm', name='My versioned cytometry dataset', version='2', hash='ZKQxIw0uAvtMtdZk8SAj', transform_id='SmQmhrhigFPLz8', run_id='Xw5xc4OteUjTU31pfcZC', initial_version_id='dwdXtuUm7KR2XuBNSbsv', created_by_id='DzTjkKse')
dataset_v2.features
hello
hello
within hello
hello
within hello
hello
within hello
hello
within hello
Features:
var: FeatureSet(id='lUT6YhM9yV4tQhh9R5Ve', n=47, type='number', registry='bionty.CellMarker', hash='KwNe7q-_6MK4WdcHsRuJ', created_by_id='DzTjkKse')
'CD14/19', 'CD8', 'Cd4', 'Ccr7', 'CD103', 'CD95', 'CD69', 'CD49B', 'CXCR4', 'CD27', 'CD45RA', 'CD3', 'CD85j', 'CD11c', 'Igd', 'CD38', 'CD16', 'CD94', 'CD20', 'CD27', ...
external: FeatureSet(id='eA6ogIIilUdLyVfVA9HN', n=2, registry='core.Feature', hash='qV1hwXGKngdbVta23Anl', updated_at=2023-10-04 16:42:41, modality_id='zBZgVlDk', created_by_id='DzTjkKse')
🔗 assay (0, bionty.ExperimentalFactor):
🔗 species (0, bionty.Species):
obs: FeatureSet(id='0FXtA0ATGyrSy7GrZ5oS', n=5, registry='core.Feature', hash='W4a3vMNiHC_k-_XfHV8F', updated_at=2023-10-04 16:42:26, modality_id='zBZgVlDk', created_by_id='DzTjkKse')
Dead (number)
(Ba138)Dd (number)
Bead (number)
Time (number)
Cell_length (number)
dataset_v2
Dataset(id='dwdXtuUm7KR2XuBNSbsm', name='My versioned cytometry dataset', version='2', hash='ZKQxIw0uAvtMtdZk8SAj', transform_id='SmQmhrhigFPLz8', run_id='Xw5xc4OteUjTU31pfcZC', initial_version_id='dwdXtuUm7KR2XuBNSbsv', created_by_id='DzTjkKse')
dataset_v2.save()
dataset_v2.labels.add(efs.fluorescence_activated_cell_sorting, features.assay)
dataset_v2.labels.add(species.human, features.species)
hello
within hello
hello
within hello
hello
within hello
hello
within hello
dataset_v2.view_flow()
hello
within hello
hello
within hello
hello
hello
hello
hello
within hello
hello
within hello
hello
hello
hello
hello
within hello
hello
within hello
hello
hello
hello
hello